Introduction
Welcome to the third installment of our Omics blog series at Arta, where we continue to explore the role of Omics technologies in cultured meat production. In our previous posts, we delved into the "why" and "where" of Omics in this industry, highlighting its potential to address complex challenges and bridge significant knowledge gaps. Now, we turn our attention to the "how" – providing a comprehensive guide on implementing Omics tools effectively in cultured meat research and development.
The journey of using Omics in cultured meat is complex and has many individual steps! It begins with the essential task of identifying key research questions, a step that sets the direction for the entire project. This foundational stage, as we've seen in our first blog, is critical for ensuring that your research is aligned with the unique challenges and opportunities in the cultured meat industry. From improving meat texture and nutritional content to reducing production costs, each goal demands a tailored Omics approach. Following this, the planning phase, as discussed in our second blog, involves selecting suitable Omics techniques, determining sample sizes, and setting clear objectives. This careful planning is crucial for the successful execution of Omics experiments, ensuring that they are not only scientifically robust but also practically relevant to the cultured meat question at hand.
In this post, we will walk you through each critical step of the Omics workflow (Figure 1), from the execution of experiments and precise sample preparation to the complex processes of data analysis and visualisation. Our focus is on providing practical, step-by-step guidance that encapsulates the depth and breadth of Omics applications in cultured meat production.
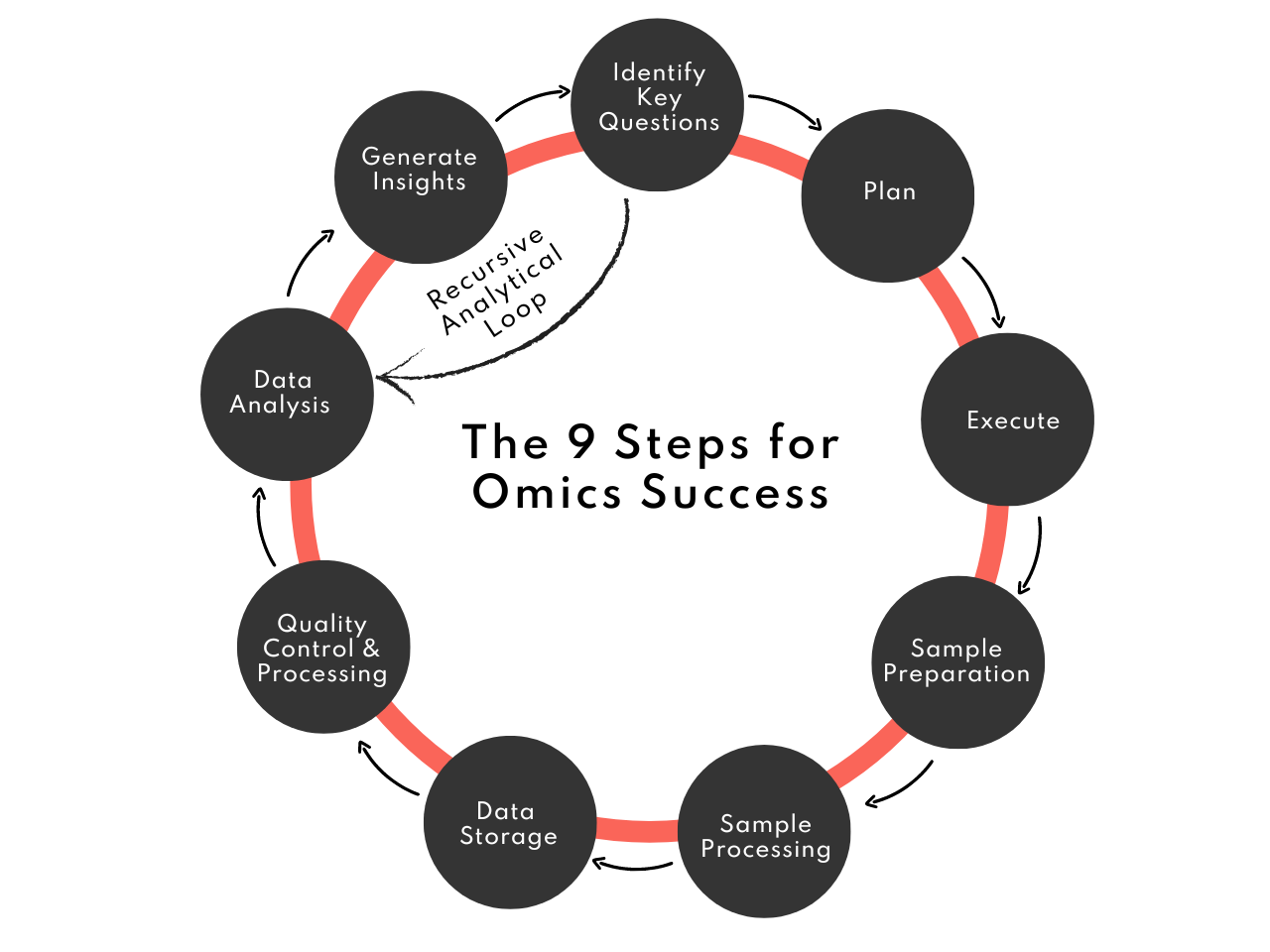
Figure 1. The 9-step Omics experimental and analytical workflow
The Process
1. Identify Key Questions
The initial stage in applying Omics technologies to cultured meat production is the clear identification of the key questions and objectives related to your research or business needs. This process is fundamentally about tailoring the Omics approach to address specific goals and challenges in the most effective way.
- Understanding Your Goals: Begin by pinpointing your primary goals. For instance, if your aim is to enhance the texture of cultured meat, your Omics experiments might focus on understanding the protein networks involved in texture formation. If improving nutritional content is the goal, then analyzing the metabolic pathways that lead to nutrient synthesis could be more relevant. Alternatively, if reducing production costs is a priority, you might investigate genetic pathways that could increase growth rates or optimise nutrient utilisation.
- Identifying Challenges: Next, it's crucial to identify the specific problems you're facing in the domain of cultured meat production. This could involve a range of issues, such as improving cell line stability for consistent meat quality, optimising growth media for better cell proliferation, or addressing regulatory requirements. For example, if cell line stability is a concern, genomic and transcriptomic analyses can be employed to study genetic stability and transcript expression stability over time.
- Setting Clear Objectives: Finally, clearly define what success looks like for your project. This involves establishing concrete, measurable objectives. If your goal is to achieve a certain growth rate, then your Omics study might concentrate on genes and proteins that regulate cell division and metabolism. For meeting specific nutritional targets, you could focus on pathways involved in the biosynthesis of essential nutrients. In the case of ensuring genetic stability, regular genomic assessments might be integrated into your workflow to monitor and maintain consistency over multiple generations.
2. Planning Your Omics Study
Once you have identified the key questions, the next crucial step is to meticulously plan your work. This involves several important considerations to ensure that the study is well-designed, feasible, and aligned with your objectives.
- Selecting the Right Omics Technique: Based on your objectives, choose the most appropriate Omics technique. For example, genomics might be ideal for understanding genetic stability, while proteomics could be better suited for studying changes in protein expression that affect meat texture. If your goal is to get a holistic view of cellular processes, a multiomics approach might be the best choice.
- Determining Sample Size and Experiment Scale: It's essential to decide on the size and scale of your experiment. This includes determining how many samples are needed to achieve statistically significant results and considering the feasibility in terms of time, resources, and costs. Larger sample sizes might provide more data, but they also require more resources and time. Generally when conducting Omics experiments, it is preferable to try to achieve a lot of output from a single experiment.
- Choosing Experimental Controls: Select appropriate controls for your experiments. Controls are crucial for providing a baseline to compare your results and for helping to ensure that the data you obtain is reliable and valid.
- Sample Preparation and Analysis: Plan how you will prepare and analyze your samples. This includes everything from cell culture conditions to the extraction and preservation of biomolecules (DNA, RNA, proteins). The methods used here must be consistent and reproducible to ensure the reliability of your data.
- Data Processing Strategy: Decide how you will process the data. This involves selecting the right software and computational tools for data analysis. If you're using a multiomics approach, consider how you will integrate data from different sources. Though this step, is less critical at this stage.
- Budget and Resource Allocation: Ensure that you have the necessary budget and resources to carry out your experiment. This includes funding for Omics technologies, laboratory materials, personnel, and data storage solutions.
- Timeline and Milestones: Develop a realistic timeline for your project, including key milestones. This helps in tracking progress and ensures that the project stays on schedule.
3. Experiment Execution
After thorough planning, the next phase is the comprehensive and detailed execution of your Omics experiment to ensure the quality and reliability of your data at the other end. This step is much like any other experiment, but for Omics work the costs are generally significantly higher, so you want to be sure that everything is done as diligently as possible to avoid the age-old experimental issue of “Shit in, Shit out”!
- Adherence to Protocols: Follow the experimental protocols developed for sample collection, handling, and sample processing. Any deviation from the planned procedure can introduce variables that may affect the reliability of your results.
- Documentation and Record-Keeping: Maintain detailed records of each step of the experiment. This includes documenting the conditions under which samples were collected, how they were processed, and any deviations from the original plan. Good record-keeping is crucial for the reproducibility of your Omics experiment and for troubleshooting any issues that might arise when the data arrives.
- Dealing with Deviations: In the case of deviations from expected experimental outcome, document them thoroughly and assess their potential impact on the results. It may be painful, but if there are significant deviations, it’s advisable to discard those samples and restart the experiment, rather than proceed. Given the substantial investment required for Omics, ensuring that the initial experimental conditions and results are optimal can prevent wasteful expenditure on flawed samples.
4. Sample Preparation
Sample preparation is a critical step in Omics research, significantly influencing the quality of your data. Proper handling and preparation of samples are essential to obtain reliable and meaningful results.
- Standardising Sample Collection: Consistency is key here. Ensure that all samples are collected under similar conditions and processed immediately or stored appropriately to prevent degradation. This is particularly important for volatile samples like RNA.
- Deciding Between In-House or Outsourcing: Consider whether to prepare samples in-house or to outsource to specialised facilities, especially for more complex or high-throughput Omics analyses. Outsourcing can offer advanced expertise and equipment but may come with additional costs and require more time for sample shipment and processing.
- Preparation Methodology: Choose the right method for preparing your samples based on the Omics technique you are using. For genomics studies, this might involve DNA extraction and purification, while proteomics studies would require protein extraction and possibly digestion. The method should be optimised to yield high-quality and sufficient quantities of the biomolecule of interest.
- Contamination Prevention: Implement measures to prevent cross-contamination between samples and contamination from external sources. This includes using clean equipment, wearing appropriate protective gear, and following strict laboratory practices.
- Use of Controls in Sample Preparation: Incorporate appropriate controls during sample preparation to validate the results. This might include using blank samples, positive controls, or spike-in controls, depending on the type of analysis being performed.
- Record-Keeping of Sample Details: Document every detail of the sample preparation process, including the type of reagents used, volumes, incubation times, and temperatures. This information is crucial for replicating the study and understanding any variations in the results.
- Quality Assessment Post-Preparation: After preparation, assess the quality of your samples, for example, checking the integrity of extracted DNA or RNA, or the purity of proteins. This step ensures that the samples are suitable for downstream Omics analyses.
5. Sample Processing
The sample processing stage is where your prepared samples are analysed using the chosen Omics tools and equipment - i.e. whether you’re using a mass spectrometer or a sequencer!
- Deciding Between In-House or Outsourcing: When it comes to the processing of Omics samples, it's often more practical and cost-effective to outsource this step to specialised service facilities. Given the complexity and the high level of technical expertise required for this, especially with advanced instruments like sequencers and mass spectrometers, most research groups and companies opt for outsourcing.
- Batching Samples for Efficiency: Organise your samples into batches for processing. This not only makes the procedure more efficient but also reduces variability. It's important to randomise samples across batches to minimise batch effects, which can skew data interpretation.
- Processing Controls Alongside Samples: Run control samples alongside your experimental samples. This is crucial for assessing the performance of the instruments and the reliability of the data being generated.
- Data Collection and Initial Checks: Once the samples are processed, collect the initial data and perform preliminary checks to ensure data integrity. This may involve looking at raw output files for anomalies or ensuring that control samples have yielded expected results.
- Handling Technical Replicates: If using technical replicates (multiple samples from the same source to check consistency), ensure they are processed and analysed consistently. Consistency in handling these replicates is key to validating the reproducibility of your results.
6. Data Transfer and Storage
The sixth step in the Omics research process is crucial for managing the large volumes of data generated. Data must be transferred and stored in a safe, secure and accessible manner, for any future processing and analysis.
- Handling Large Data Volumes: Omics technologies, especially genomics and transcriptomics, often generate vast amounts of data. Efficient methods for transferring this data from the instruments to analysis servers or storage systems are essential. This might involve using high-speed data transfer protocols, cloud-based services or physical storage devices, depending on the size of the data and available infrastructure.
- Data Storage Solutions: Choose appropriate storage solutions based on the size and sensitivity of your data. For short-term needs or active analysis, local servers or high-performance computing environments may be suitable. For long-term storage, cloud-based services offer scalability and often have robust data security measures in place.
- Organising Data for Accessibility: Organise your data in a logical and accessible manner. This includes proper labeling, creating directories or folders for different projects or experimental runs, and maintaining detailed metadata. Good organisation is crucial for efficient data retrieval and analysis.
- Data Format and Compatibility: Ensure that the data is stored in formats compatible with your planned analysis tools. Some analysis software may require specific data formats, so it’s important to convert and store your data accordingly.
- Documentation of Storage Practices: Document your data storage and transfer procedures. This documentation should include details on how data is transferred, where it is stored, the formats used, and any security measures in place. This is important for reproducibility and for sharing data with collaborators or for publication purposes.
7. Quality Control and Data Processing
The next step is focused on ensuring the quality of the data obtained and processing it for analysis. Data quality control is a key part of ensuring reliability throughout the rest of your analytical pipeline, and is very easy to overlook.
- Initial Data Quality Assessment: Begin by conducting an initial quality assessment of the raw Omics data. This may involve checking for any anomalies or inconsistencies in the data, such as peak resolution or signal intensity in mass spectrometry data or read quality and distribution in sequencing data. Tools specifically designed for quality control in Omics data can be used for this purpose.
- Data Normalisation and Standardisation: Apply appropriate normalisation techniques to reduce biases and variability that might be present in the data. This step is particularly important when comparing datasets from different batches, experiments, or Omics platforms. Normalisation ensures that the data can be directly compared and accurately interpreted.
- Data Cleaning and Pre-processing: Clean the data by removing background noise, filtering out low-quality or irrelevant data points, and correcting any detectable errors. This might involve trimming sequences, aligning reads in genomic data, or calibrating mass spectrometry data.
- Documentation of Data Processing Steps: Document every data processing step meticulously. This includes the software and algorithms used, parameters set, and any decisions made during the processing. This documentation is vital for the reproducibility of the study and for future reference.
- Preparing Data for Analysis: Finally, format and structure the processed data in a way that it is ready for subsequent analysis. This might involve organising data into matrices or databases, labelling, and ensuring that the data is compatible with the analysis tools you plan to use.
8. Data Analysis and Visualisation
The eighth step in the Omics workflow involves the critical tasks of analyzing the processed data and visualizing the results. This phase transforms raw data into understandable and interpretable information, unveiling the biological insights hidden within.
- Selecting Analysis Tools: Choose the appropriate bioinformatics tools and software for data analysis. The selection depends on the type of Omics data and the specific questions being addressed. For instance, different tools will be used for analysing transcriptomic data compared to proteomic data. Ensure the tools are well-suited to handle the complexity and volume of your data.
- Performing Statistical Analysis: Conduct rigorous statistical analyses to identify significant patterns, differences, or correlations in the data. This may include differential expression analysis, pathway analysis, or statistical tests to compare different conditions or groups. Ensure that the statistical methods are appropriate for the data type and the hypotheses being tested.
- Data Visualisation: Visualise the data in a way that highlights key findings and is easily interpretable. This might involve generating graphs, heat maps, or network diagrams. Good visualisation helps in understanding complex data sets and communicating results to both scientific and non-scientific audiences.
- Identifying Patterns and Trends: Look for patterns, trends, and anomalies in the data. This step is crucial for generating hypotheses, understanding biological mechanisms, and identifying potential targets for further investigation.
- Iterative Analysis Process: Data analysis is often an iterative process. Based on initial findings, you may need to go back and refine your analysis, adjust parameters, or even revisit earlier steps in the workflow. This iterative approach ensures a thorough exploration of the data.
- Documenting the Analysis: Document all analysis procedures, including the software used, parameters set, and the rationale behind methodological choices. This documentation is essential for the reproducibility of your results and for future reference.
9. Insight Generation
The final stage of the Omics workflow is the generation of insights from data, where the analyzed data is interpreted to extract meaningful and actionable information. This step bridges the gap between data analysis and practical applications, and it marries the data science from earlier steps with deep biological knowledge and intuition.
- Interpreting Results: This involves understanding what the data is indicating in the context of your initial questions and hypotheses, it's about translating the statistical findings into actionable biological significance.
- Drawing Conclusions: Based on the analysis, draw conclusions about the state of the biological systems or process. For instance, you might infer which genetic pathways are crucial for muscle tissue development in cultured meat, or which metabolic processes are essential for nutrient synthesis.
- Relating Findings to Cultured Meat Objectives: Connect your findings back to the specific objectives and challenges of cultured meat production. This could involve identifying potential improvements in production methods, uncovering new insights into cell growth and development, or revealing factors that influence meat quality and taste.
- Identifying Practical Next Steps: Look for practical applications of your findings. This might include developing new strategies for cell line selection, optimising growth media, or identifying targets for genetic modification to enhance meat quality.
- Communicating Findings: Clear and concise communication of data-generated insights is crucial for advancing cultured meat production, raising awareness and raising capital.
- Documentation for Future Reference: Finally, document your insights and conclusions in a detailed manner. This record should include how the insights were derived, their implications, and potential limitations or areas for further investigation.
Recursive Analytical Loop: Steps 8, 9, and 1
In the Omics research process, especially in complex fields like cultured meat production, a recursive analytical loop often emerges, linking the stages of Data Analysis and Visualization (Step 8) and Insight Generation (Step 9) back to the initial stage of Identifying Key Questions (Step 1). This iterative cycle is vital for deepening understanding and refining the questions asked of the data
- From Insight to New Questions: As insights are generated in Step 9, they often raise new questions or highlight areas that need further exploration. These new questions or areas of interest are not endpoint conclusions but rather springboards for additional inquiry. They direct the focus back to Step 1, where new or refined questions are formulated based on the latest findings.
- Revisiting Data Analysis with Fresh Perspectives: With new questions in hand, researchers return to Step 8, where the existing data can be re-analysed or viewed from a different perspective, or where the realisation emerges that additional data may be needed. This revisiting of data analysis is not merely a repetition; it's an opportunity to dig deeper, apply new analytical techniques, and focus on previously overlooked aspects of the data.
- Expanding the Scope of Questioning: Over time, this recursive loop can lead to an expansion of the research scope. New questions may lead to broader or entirely different areas of investigation within cultured meat, driving innovation and discovery in the field.
Conclusion
In wrapping up our exploration of Omics technologies in cultured meat production for now, we've seen how these tools can be methodically applied to address various aspects of this field. From framing the right research questions to the detailed analysis and interpretation of data, Omics offers a comprehensive approach to tackling a large number of challenges in cultured meat. The steps outlined in this series provide a roadmap for integrating Omics into your research or production processes.
If you're considering how Omics can enhance your work in cultured meat or if you have specific questions about applying these technologies, we at Arta are here to help. Our team is equipped to support your Omic journey in cellular agriculture, offering tailored advice and solutions.
Thank you for following this mini-series on Omics in cultured meat! For further information or to discuss how we can assist you in applying Omics in your projects, feel free to reach out to me at alex@artabioanalytics.com.
Comments
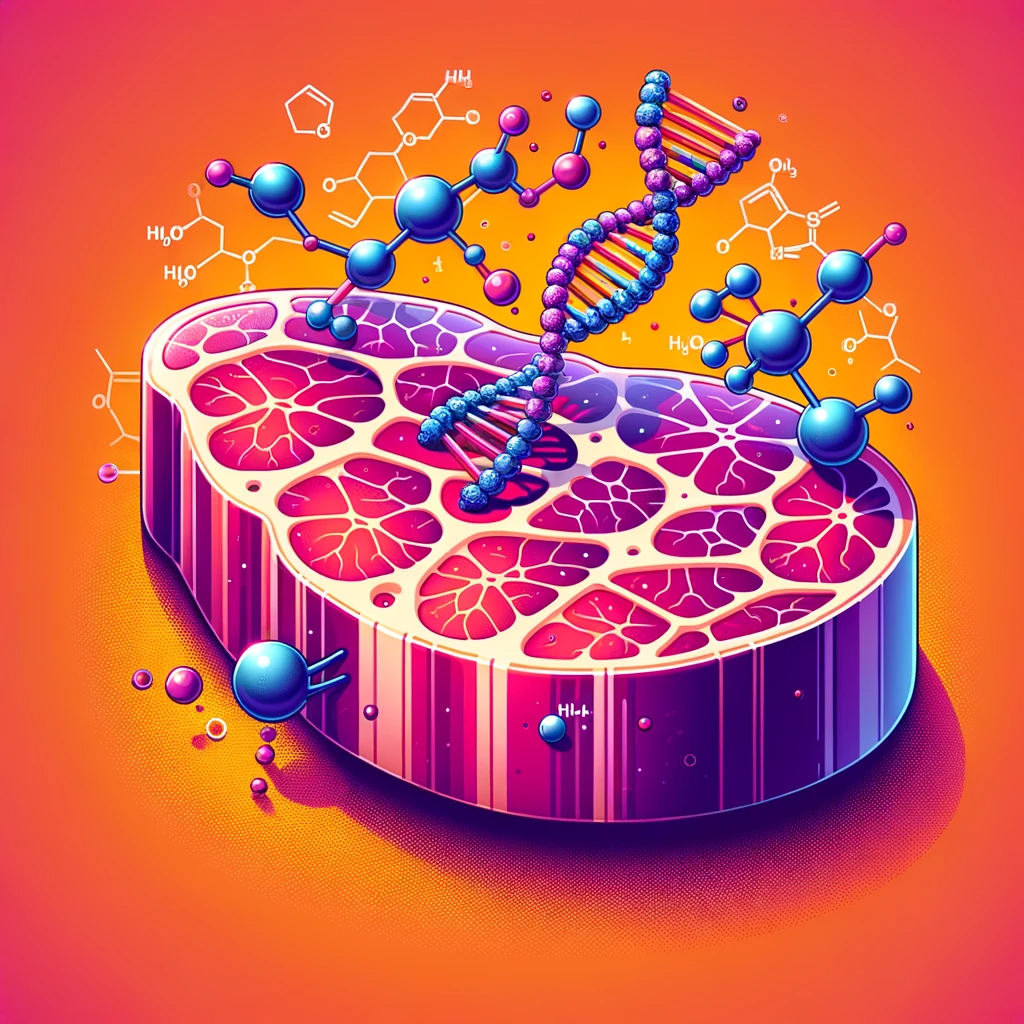